Live Production
Live Production
IBC Show VOD
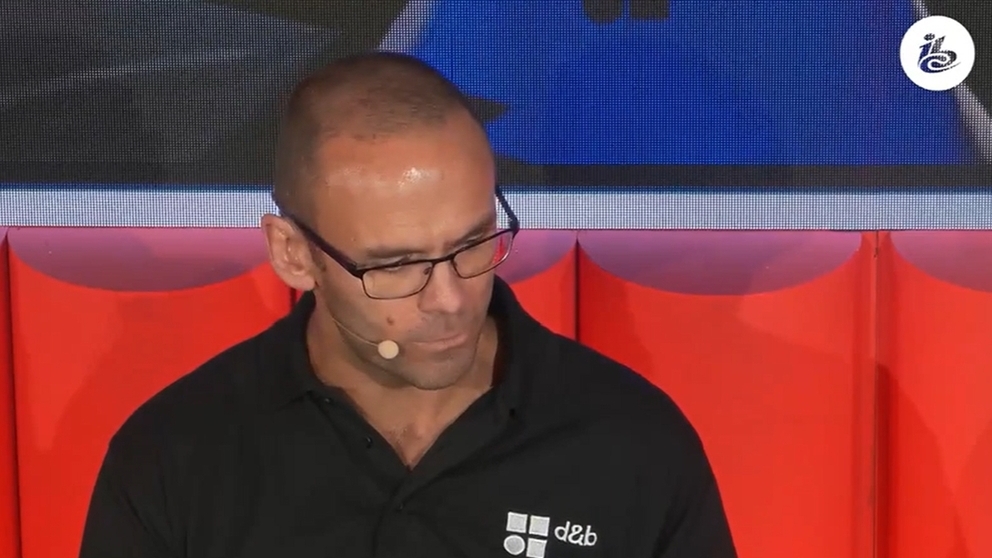
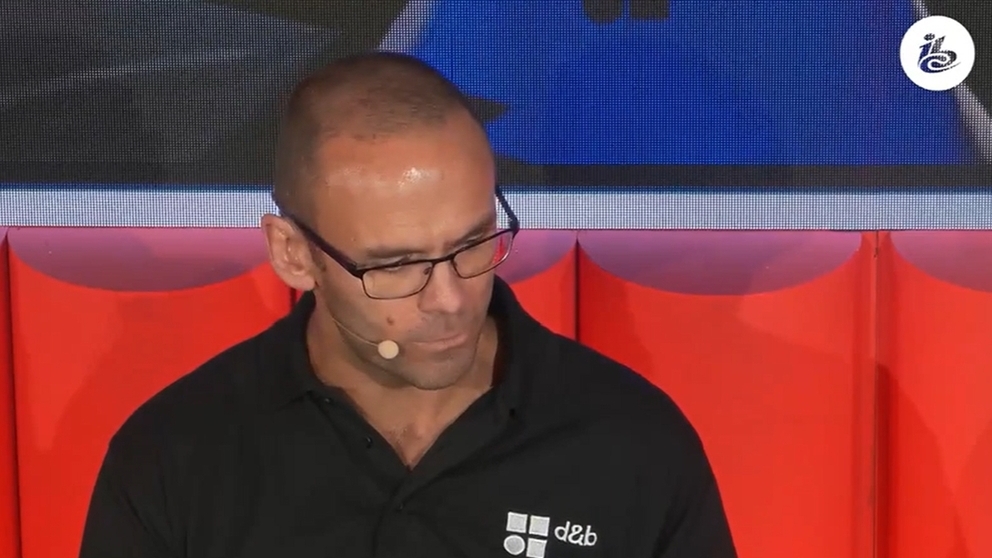
IBC Accelerators: “Seeing it work and seeing the future possibilities for Live Performances made it all worthwhile”
IBC Show VOD

Media Production without Boundaries: “There’s a lot of cool stuff we could do but why should we do it.”
IBC Show VOD


Media Production without Boundaries: We need to pull together to make these ‘interesting times’ work
IBC Show VOD


Accelerators - Evolution of the Control Room: helping content creators to produce a show “without advanced technical expertise”
IBC Show VOD


From Paris to Hong Kong: How LTN and IMAX stream the biggest live events to global audiences with theatre-grade quality
IBC Show VOD

